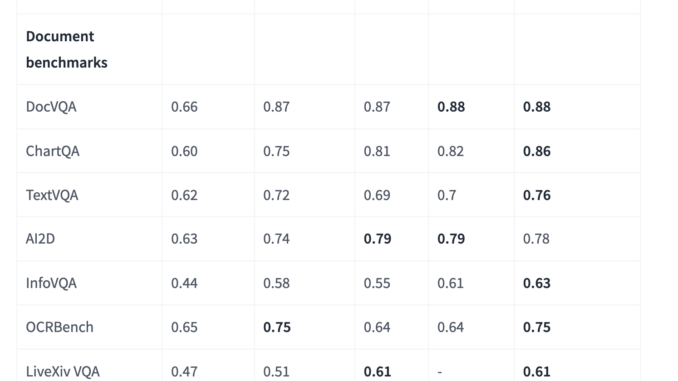
The integration of visual and textual data in artificial intelligence presents a complex challenge. Traditional models often struggle to interpret structured visual documents such as tables, charts, infographics, and diagrams with precision. This limitation affects automated content extraction and comprehension, which are crucial for applications in data analysis, information retrieval, and decision-making. As organizations increasingly rely on AI-driven insights, the need for models capable of effectively processing both visual and textual information has grown significantly.
IBM has addressed this challenge with the release of Granite-Vision-3.1-2B, a compact vision-language model designed for document understanding. This model is capable of extracting content from diverse visual formats, including tables, charts, and diagrams. Trained on a well-curated dataset comprising both public and synthetic sources, it is designed to handle a broad range of document-related tasks. Fine-tuned from a Granite large language model, Granite-Vision-3.1-2B integrates image and text modalities to improve its interpretative capabilities, making it suitable for various practical applications.
The model consists of three key components:
Vision Encoder: Uses SigLIP to process and encode visual data efficiently.
Vision-Language Connector: A two-layer multilayer perceptron (MLP) with GELU activation functions, designed to bridge visual and textual information.
Large Language Model: Built upon Granite-3.1-2B-Instruct, featuring a 128k context length for handling complex and extensive inputs.
The training process builds on LlaVA and incorporates multi-layer encoder features, along with a denser grid resolution in AnyRes. These enhancements improve the model’s ability to understand detailed visual content. This architecture allows the model to perform various visual document tasks, such as analyzing tables and charts, executing optical character recognition (OCR), and answering document-based queries with greater accuracy.
Evaluations indicate that Granite-Vision-3.1-2B performs well across multiple benchmarks, particularly in document understanding. For example, it achieved a score of 0.86 on the ChartQA benchmark, surpassing other models within the 1B-4B parameter range. On the TextVQA benchmark, it attained a score of 0.76, demonstrating strong performance in interpreting and responding to questions based on textual information embedded in images. These results highlight the model’s potential for enterprise applications requiring precise visual and textual data processing.
IBM’s Granite-Vision-3.1-2B represents a notable advancement in vision-language models, offering a well-balanced approach to visual document understanding. Its architecture and training methodology allow it to efficiently interpret and analyze complex visual and textual data. With native support for transformers and vLLM, the model is adaptable to various use cases and can be deployed in cloud-based environments such as Colab T4. This accessibility makes it a practical tool for researchers and professionals looking to enhance AI-driven document processing capabilities.
Check out the ibm-granite/granite-vision-3.1-2b-preview and ibm-granite/granite-3.1-2b-instruct. All credit for this research goes to the researchers of this project. Also, don’t forget to follow us on Twitter and join our Telegram Channel and LinkedIn Group. Don’t Forget to join our 75k+ ML SubReddit.
🚨 Recommended Open-Source AI Platform: ‘IntellAgent is a An Open-Source Multi-Agent Framework to Evaluate Complex Conversational AI System’ (Promoted)
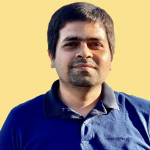
Asif Razzaq is the CEO of Marktechpost Media Inc.. As a visionary entrepreneur and engineer, Asif is committed to harnessing the potential of Artificial Intelligence for social good. His most recent endeavor is the launch of an Artificial Intelligence Media Platform, Marktechpost, which stands out for its in-depth coverage of machine learning and deep learning news that is both technically sound and easily understandable by a wide audience. The platform boasts of over 2 million monthly views, illustrating its popularity among audiences.
Be the first to comment